Using Perplexity.ai's Spaces as a life raft in an age of AI slop
It's well-known at this point how poor traditional search engines have gotten. That was before Large Language Model (LLM)-generated slop flooded the field. Which means it's weird to see me talking about a good use case for LLMs if you've only seen and heard about the bad. I'll hit on that in a future post, maybe. For now: the good stuff!
First: the elephant in the room.
"But what about the environmental cost?" you sagely ask.
So here's the thing: Google invented transformers. That's the paper and technology behind LLMs. They've been using this stuff and its predecessor technologies since the day it was founded. All search engines do.
The ethical issues regarding scraping and citation for LLM-based search are the same ones we collectively debated at length when web search engines first emerged and I'm not sure we ever arrived at a good answer. Debates and lawsuits continue over it.
I don't know if LLMs are worse on energy use than other machine learning-based technologies search engines use, but LLMs are in the mix, and they're here to stay. So why not search less?
What if you could drop a couple of words into a box and have all, or most, of your questions on a topic addressed and maybe even fully answered in an outline?
What if you could do this instead of pleading with Google or Bing or DuckDuckGo for 30, 50, 100 queries trying to find that nugget buried under all the slop churned out by SEO dipshits? (not to be confused with garden variety SEO focused on making pages friendly to both humans and robots)
Welcome to the world where you can do that. It's already here! Neat.
You know how every search engine under the sun is putting an "Answers" thing in there? That's them recognizing the problem and trying to solve it with LLMs. Most of them use a model that they make publicly available for others to use, or use one of those models.
Enter: Perplexity.
Perplexity is an LLM-based–"AI-based" if you must–search engine with a focus on applying these popular models to research. It blows ChatGPT out of the water for pretty much every prompt involving looking stuff up and summarizing. At least in my experience. And it would be unfair to compare it to traditional search engines.
LLM models are trained on all that stuff you try to dig through in traditional search engines, plus the good stuff, and more. They go through a process of human and machine-mediated refinement to get rid of the noise. The exact how seems to change monthly, and it's often secret and proprietary, but the gist is they keep getting better.
Cloud-based LLM search things employ something called Retrieval-Augmented Generation, or RAG for short. This lets a system combine current information with a model that might have seen its last data a year or three ago. You can do this with local LLMs, but enabling RAG on local models is more complicated and out of scope for this article. It also introduces some fun security considerations you have to think about and understand!
All the cloud-based LLM things do search. Perplexity takes it a step further with Spaces. Here's a screenshot of my "The Meta" space in action.
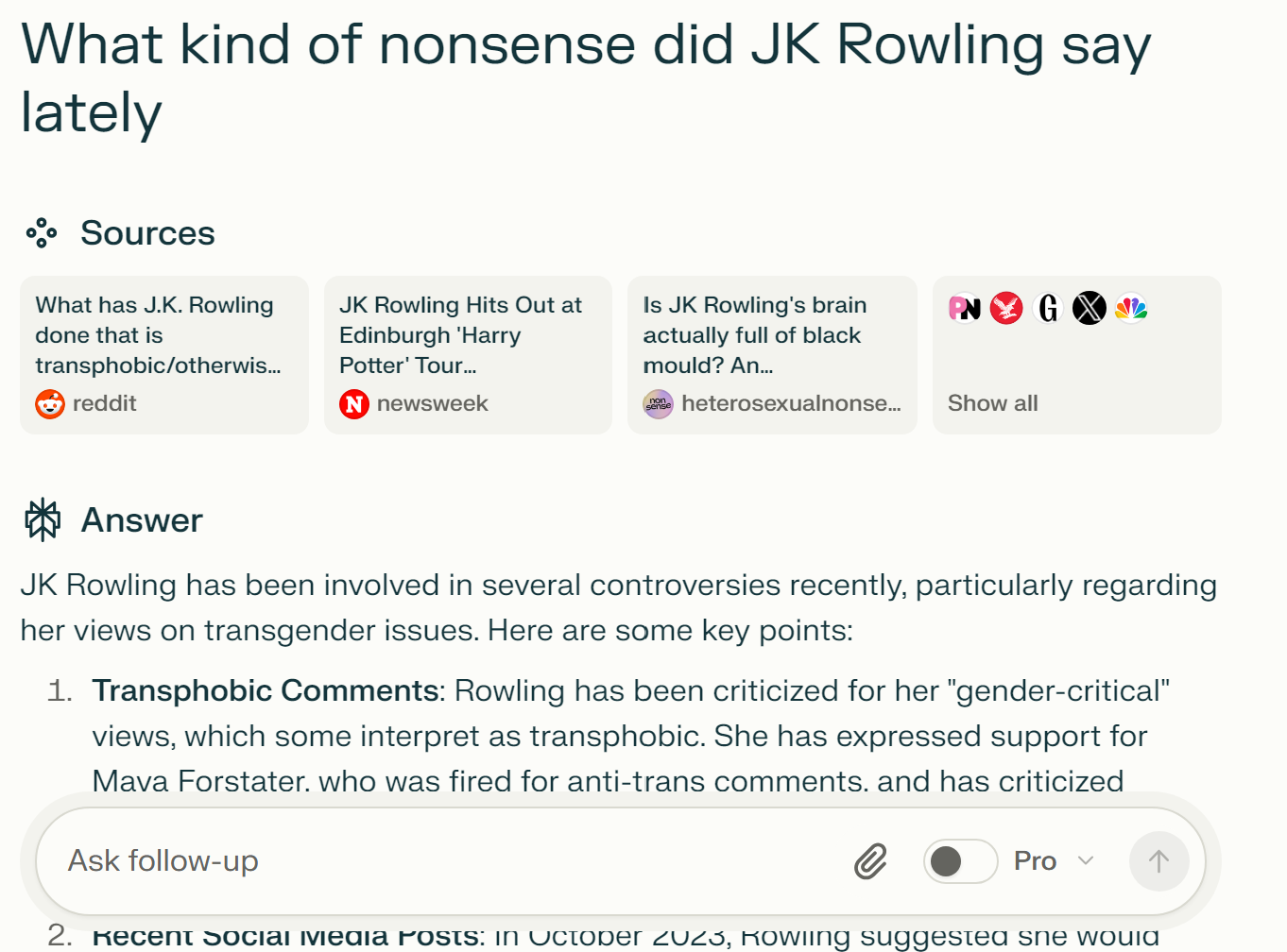
You basically have it start with a premise about what a space is for. That way you can just drop a link or a phrase and have it do the thing you want it to do without prompting. All the threads produced by this prompt are in the space. Follow-ups are limited on the free plan, but it usually gets everything right on the first try. If it doesn't, that's usually a sign the space's prompt needs work!
Thread Summaries prompt: "Always summarize the linked discussion thread with credited quotes reflecting the different perspectives. Attach the permalink from the quoted comment to the quote in the summary. Include a list of any links with the name of the commentor who shared them."
I've tried to just stop reading Reddit, Hacker News, etc but I do occasionally find something useful there. This space grinds it all down and pans the gold for me. The links to posts don't currently work, but it does provide the user names. I can page search those on Hacker News, and it's usually in the latest comments on Reddit user pages. I have feedback in to Perplexity, so this should work better in the future.
The Gist prompt: "Always respond to links with a summary and outline."
Some people hate spoilers. I don't. And with non-fiction, I find knowing what I'm about to read helps with recall and understanding. I only do this for news and educational type stuff, not personal essays.
The Meta prompt: "Topics I ask about here are something I've heard about but can't find context on. Usually current events. Look up the topic and see what the latest news and discussion is about. Add context if it's something ongoing."
The Meta is mainly used when someone vagueposts on social media. I can drop some odious figure's name in and see what kind of nonsense they said that has everyone talking so I can avoid a bunch of stressful scrolling and searching to figure it out. If a recurring pain in the butt hasn't risen to the level of recent news articles, there's probably nothing new or urgent. I can also drop trending topics in here and completely short-circuit any urge to doomscroll.
That's all I have for now, because these three spaces collectively handle everything I've thrown at them. I haven't created a special purpose space for doing research on a topic yet, but that's an obvious next step.